AI's Role in Contextual Data Processing
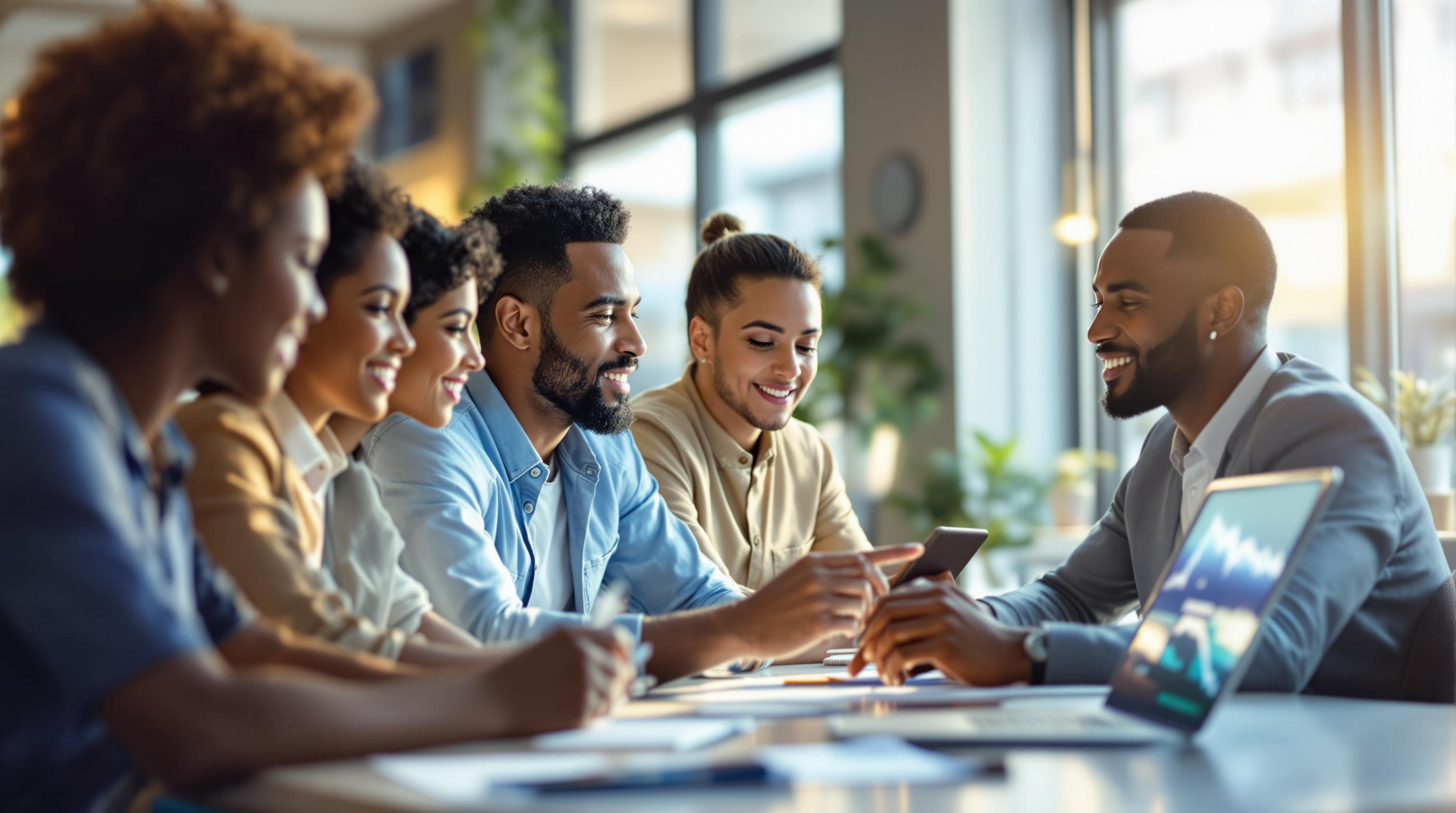
AI is changing how data is understood by focusing on context - like time, location, and user behavior. Instead of treating data as isolated points, AI connects the dots, especially when dealing with unstructured data like images, documents, and social media. This leads to smarter decisions across industries:
Healthcare: Combines patient history, real-time vitals, and demographics for better diagnosis.
Finance: Detects fraud by analyzing patterns in transactions and user behavior.
Manufacturing: Uses real-time and historical data to optimize operations.
AI’s ability to analyze context improves risk assessments, predictions, and personalization. For example, fitness apps recommend workouts based on weather, location, and schedule. However, success depends on balancing technological advances with ethics and privacy.
Key advancements include real-time analysis, better anomaly detection, and tools like BestofAI, which offers access to over 12,592 AI tools for various needs. The future of contextual AI lies in managing diverse data, ensuring privacy, and delivering actionable insights responsibly.
Related video from YouTube
Why Context is Important in Data Processing
Context provides the background and relationships needed to transform raw data into useful insights. In today’s data-heavy world, understanding the circumstances surrounding data points is essential for making smarter decisions.
Take customer behavior, for example. Context like holiday seasons, marketing campaigns, or price fluctuations can reveal trends and patterns that help businesses create better strategies. This approach ensures decisions are grounded in data that reflects the bigger picture.
There are four main dimensions where context plays a role in how organizations get value from their data:
Dimension | How It Shapes Data Processing |
Technical | Impacts how data is gathered, processed, and analyzed |
Humanistic | Reflects user behavior, preferences, and social influences |
Ethical | Ensures responsible data use and privacy protection |
Economic | Drives business value and shapes decision-making |
When dealing with unstructured data like images or videos, context becomes even more critical. These formats don’t follow a set structure, so understanding the surrounding elements is key to pulling out meaningful insights. AI tools are particularly good at analyzing these diverse data types by factoring in context.
Systems that incorporate context - like fitness or travel apps - become far more accurate and relevant. For example, a fitness app might recommend workouts based on the weather, your schedule, location, or even what your friends are doing. This makes the recommendations much more personalized and useful.
Context is also a game-changer for risk assessment and forecasting. By layering in multiple contextual factors, businesses can uncover patterns, flag unusual activity, and make predictions with greater accuracy.
What is Contextual Data Processing?
Contextual data processing brings external factors into data analysis, going beyond raw numbers to uncover deeper insights. It looks at dimensions like time, location, user behavior, and external events to create a fuller picture.
"Context is essential in applying data science and AI." - Spector et al., 2022
In healthcare, this approach helps doctors make better diagnoses by factoring in patient history, lifestyle, and environmental conditions along with current symptoms. For example, when processing patient data, the system might consider seasonal trends, demographic details, and local health patterns to suggest more accurate treatments.
The strength of contextual data processing lies in its ability to fill gaps in incomplete data and enhance decision-making. Here's a look at how it's applied across various industries:
Industry | Application | Impact |
Healthcare | Patient monitoring and diagnosis | Combines real-time vitals with patient history and external factors |
Finance | Risk assessment and fraud detection | Evaluates transactions alongside user behavior and market conditions |
Cybersecurity | Threat detection | Analyzes alerts with network activity and global threat intelligence |
Modern systems use AI to handle unstructured data, uncovering patterns and connections that traditional methods might miss. In cybersecurity, for instance, contextual processing can spot unusual activity by examining not just a single alert, but also the user's behavior, time of access, and global threat data.
AI has transformed contextual data processing from rigid rule-based systems to flexible, learning-driven models. These systems make smarter, context-aware decisions that align with the complexities of real-world scenarios. With AI's ability to adapt and evolve, contextual processing is reshaping how industries approach decision-making.
How AI is Changing Contextual Data Processing
AI is transforming the way contextual data is processed, allowing systems to handle various data types while considering factors like time and location. This evolution has taken organizations from rigid, rule-based systems to advanced learning models that provide deeper and more actionable insights.
Here’s how AI is reshaping contextual data processing across three main areas:
Aspect | Traditional Methods | AI-Powered Methods |
Speed | Relied on manual reviews and batch processing | Enables real-time analysis with instant insights |
Scope | Focused only on structured data | Works seamlessly with both structured and unstructured data |
Context Integration | Dependent on fixed rules and parameters | Adapts dynamically through continuous learning |
AI’s ability to process massive datasets while staying context-aware has greatly improved both accuracy and efficiency. A great example of this is in retail: AI can analyze customer behavior, factoring in location and time, to make real-time inventory decisions. This allows stores to predict demand trends and adjust stock levels accordingly.
By evaluating multiple layers of context at once, AI uncovers subtle patterns and relationships that might elude human analysts. This deeper understanding enables businesses to predict trends and act proactively. For instance, AI can identify emerging market trends by analyzing a mix of social media sentiment, news articles, and economic data. This approach provides a more nuanced perspective than traditional analysis methods.
For companies looking to adopt AI-driven contextual processing, platforms like BestofAI offer access to over 12,592 specialized AI tools across different categories. These resources make it easier for businesses to find and implement the right solutions for their unique requirements.
These innovations are paving the way for real-time context analysis, revolutionizing how industries operate and make decisions.
Key Parts of Contextual Data Processing
Contextual data processing depends on four main components that work together to extract useful insights from complex data sets. Each part plays a key role in helping AI systems interpret and process information within its context.
Understanding Data Through Meaning and Metadata
AI uses semantic maps to organize unstructured data, helping it recognize the relationships between different pieces of information. This approach allows systems to grasp not just the literal content of the data but also its broader significance. For example, when analyzing medical records, AI doesn’t just scan the text - it identifies connections between symptoms, treatments, and outcomes.
Analyzing Behavior and Social Connections
Behavioral analysis examines patterns in user actions and social networks. AI can spot subtle changes in behavior that may signal important trends or shifts. This is especially useful in areas like fraud prevention and improving customer experiences.
Analysis Type | Purpose | Key Benefits |
Pattern Recognition | Detects recurring behaviors/anomalies | Helps anticipate and respond proactively |
Social Network Analysis | Maps relationships and influences | Uncovers hidden links and trends |
Sentiment Analysis | Measures emotional tone and reactions | Offers insights into user attitudes |
While understanding behavior and social ties provides valuable insights, adding time and location data deepens the context for analyzing information.
Time and Location Context
The inclusion of time and location data adds another layer of meaning to the information being processed. For instance, delivery companies rely on this data to optimize routes and predict potential delays. This type of context is critical for applications like supply chain management and personalized services, improving decision-making accuracy across different scenarios.
AI Methods for Processing
To handle contextual data, AI employs tools like natural language processing (NLP), machine learning, deep learning, and neural networks. These methods enable systems to learn, identify patterns, and map relationships in ways that mimic how humans understand context.
"The importance of context in data science and AI cannot be overstated. It's what transforms raw data into actionable insights that drive better decision-making across organizations", highlights a recent industry study.
Understanding Data Through Meaning and Metadata
The use of large language models (LLMs) has transformed how we process documents, offering a deeper grasp of context and meaning. These systems can now interpret subtle nuances and fix errors automatically, streamlining data processing and improving accuracy.
Today’s AI doesn’t just rely on basic keyword matching. Instead, it analyzes complex connections between pieces of information. This shift has changed how organizations handle and understand massive amounts of unstructured data, focusing on both meaning and metadata.
Data Type | Processing Method | Key Benefits |
Unstructured Text | Natural Language Processing | Understands context and extracts meaning |
Visual & Metadata Content | Computer Vision & Semantic Analysis | Detects patterns and uncovers data relationships |
Metadata plays a crucial role by tying together contextual elements like timestamps or user activity, enabling systems to deliver insights in real time. This approach improves pattern recognition and enhances predictions.
"AI-driven data processing must address ethical and privacy challenges to ensure compliance with societal norms", explains a study published in the Journal of Big Data.
In healthcare, AI systems analyze data patterns to support more accurate and informed decisions. By combining explicit medical data with contextual insights, these tools enhance patient care, taking into account individual needs and external factors.
Platforms like BestofAI provide access to over 12,592 tools, empowering organizations to adopt AI for tasks such as document analysis and semantic understanding.
Analyzing Behavior and Social Connections
AI systems are changing the way organizations understand behavior and social networks. By combining multiple layers of context, these systems create detailed user profiles and map out complex relationships. This is done through various analytical approaches, each designed to meet specific business objectives.
These systems look at direct interactions and shared behavioral patterns across digital platforms. By studying these patterns, AI can uncover meaningful relationships and even predict future actions with growing precision.
Analysis Type | Key Insights and Impact |
Behavioral Analysis | Tracks user actions and preferences, enabling personalized recommendations and fraud detection. |
Social Network Mapping | Examines network connections to detect communities and predict trends. |
Pattern Recognition | Uses historical data and group dynamics for risk assessment and market segmentation. |
For instance, retail platforms often combine behavioral analysis with social network mapping to spot influencer trends and predict which products will become popular. AI pulls data from various sources - like social media and customer interactions - to create these detailed profiles.
This ability to understand group dynamics is particularly useful in market research. By analyzing social connections, businesses can identify trends and consumer preferences before they hit the mainstream.
When behavioral insights are paired with factors like time and location, AI systems can offer even more precise and actionable recommendations.
"Context is essential in applying data science and AI, just as it is in most fields: for example, from political philosophy to engineering to statistics." - Spector et al., 2022, Data Science in Context: Foundations, Challenges, and Opportunities
Using Time and Location in Data Processing
AI leverages time-based data to uncover patterns and trends that develop over periods, while location-based processing examines geographic relationships to provide deeper understanding. When combined, these approaches allow AI systems to deliver highly accurate, context-sensitive solutions.
Contextual Element | Business Impact | Application Example |
Temporal Analysis | Detects patterns and seasonal trends | Predicting retail demand using past sales data |
Location Intelligence | Improves geographic decision-making | Enhancing supply chain efficiency through route optimization |
Combined Analysis | Delivers a richer understanding of context | Evaluating financial risks in transactions |
This fusion of time and location data has brought significant advancements to disaster management. For instance, during natural disasters, AI systems process live weather updates, track population movements, and assess infrastructure conditions. This enables them to streamline emergency resource allocation and design evacuation plans. These systems can even adjust their recommendations in real time as new data becomes available.
However, organizations implementing time and location-based data processing must address privacy concerns and adhere to ethical standards. Transparency about data collection and strict compliance with local regulations are essential to balance privacy with effective contextual analysis.
AI's ability to handle temporal and spatial data has transformed the way organizations approach context-sensitive tasks. These systems can simultaneously analyze patterns across different time zones and locations, making real-time adjustments to reflect changes in both dimensions.
AI Methods for Better Contextual Data Processing
AI-driven contextual processing is particularly effective at managing unstructured data, which forms a large chunk of business information today. By leveraging advanced techniques, AI transforms raw, unorganized data into actionable insights. This is achieved through a combination of machine learning, natural language processing (NLP), and computer vision, which work together to uncover complex patterns that older systems often miss.
These methods allow AI systems to identify patterns, interpret text, and analyze visuals - all while keeping the context intact. For instance, NLP powers chatbots in customer service, enabling them to understand inquiries, maintain the flow of conversation, and respond based on prior interactions and customer history.
AI Method | Primary Function | Key Benefit |
Machine Learning | Pattern Recognition | Automatically adjusts to new data patterns |
Natural Language Processing | Text Understanding | Derives meaning from human language |
Computer Vision | Visual Analysis | Interprets images and videos with context |
Deep Learning | Complex Pattern Analysis | Handles intricate, multi-dimensional data |
By integrating these techniques, organizations can process data more efficiently without losing contextual relevance. For example, in manufacturing, AI can simultaneously analyze sensor readings, maintenance logs, and operational data to predict equipment breakdowns before they happen.
The success of AI largely hinges on its ability to understand relationships between different data points. Advanced algorithms ensure that these connections are preserved across various data types, keeping the insights both relevant and useful. Choosing the right mix of AI methods is crucial and should align with specific business goals, factoring in the type and amount of data being processed.
These methods set the stage for real-time contextual analysis, which will be discussed in the next section.
Real-Time Analysis of Context
Real-time contextual analysis has transformed how AI handles and interprets data as it comes in. Unlike older batch processing methods, today’s AI systems can analyze data streams continuously, keeping the context accurate and relevant.
By pulling together information from various sources in real time, these systems create a clearer picture of what's happening. AI can pick up on subtle patterns and connections that might go unnoticed by humans, especially when dealing with fast-moving data.
Take manufacturing as an example. AI systems combine sensor data, equipment updates, and operational metrics to deliver instant insights. This helps facility managers catch potential problems early - before they turn into expensive breakdowns.
Analysis Type | Processing Speed | Key Application |
Streaming Data | Milliseconds | Equipment monitoring |
Behavioral Patterns | Seconds | User activity tracking |
Real-time analysis is particularly useful in situations where quick decisions are essential. To do this effectively, AI must keep track of context over different timeframes. It compares short-term patterns with historical data while factoring in current conditions. This ability to "think" across time helps AI make more accurate predictions and provide better decision-making support.
The system doesn’t just stop at one analysis - it continuously updates its understanding as new data comes in. This ensures that its insights stay relevant, even as situations change. This flexibility is especially important in fast-paced scenarios, like disaster response. In such cases, AI processes weather updates, tracks population movements, and evaluates resource availability all at once to assist emergency teams.
As this field advances, its role in identifying anomalies and improving predictive modeling will only grow more important.
Detecting Anomalies and Predicting Outcomes
AI has made huge strides in identifying anomalies and predicting outcomes by analyzing contextual data. This allows it to spot unusual events and forecast scenarios across various areas with greater precision.
When it comes to detecting anomalies, AI doesn't just rely on static thresholds. Instead, it evaluates data patterns against established benchmarks while factoring in the context - such as time-based trends, user behaviors, or external conditions. For example, a spike in activity during a seasonal sale or maintenance downtime might look unusual at first glance but could actually be expected in that context. AI ensures these nuances are taken into account.
The introduction of Large Language Models (LLMs) has further boosted these capabilities, especially when dealing with unstructured data like text. These models can identify subtle deviations in language patterns while staying tuned to industry-specific contexts. This makes them more adaptable than traditional systems, which often fail to capture these fine-grained irregularities.
Detection Type | Description and Application |
Pattern-based | Relies on historical trends and seasonal data for tasks like fraud detection. |
Behavioral | Monitors user actions to enhance security measures. |
Environmental | Considers external conditions, such as weather, for equipment maintenance. |
AI takes this a step further by layering multiple contextual factors to produce tailored insights. Take fitness recommendation systems, for instance:
"The integration of context-aware artificial intelligence algorithms in fitness recommender systems has demonstrated significant improvements in recommendation efficiency by incorporating contextual information such as user behavior and location." - Venkatachalam Parvathy and Ray Sanjog, 2022
Another area where AI shines is in spotting trends early. By analyzing unstructured data - like text, images, or sensor readings - it can detect early warning signs of problems or opportunities. This allows organizations to act before issues escalate or to seize opportunities faster than competitors.
What makes modern AI stand out is its ability to maintain context across different data types and timeframes. Instead of looking at data points in isolation, AI connects the dots, providing insights that are both accurate and practical. This interconnected approach leads to smarter decisions and better outcomes.
With these advancements, AI is becoming a powerful tool for delivering real-time, actionable insights across a range of industries.
Working with Unstructured Data
AI has reached new heights in processing unstructured data - information that doesn't fit neatly into rows and columns of traditional databases. With advanced capabilities, it can now make sense of text, images, audio, and other types of content while keeping their context intact.
What sets modern AI apart is its ability to connect the dots across various data types. Take a medical record, for example: AI can analyze written notes, lab results, and medical images simultaneously, recognizing how they relate to one another. This interconnected analysis provides insights that wouldn't be possible by examining each piece separately.
Data Type | Applications |
Text & Documents | Identifying risks, analyzing contracts |
Images/Video | Understanding scenes, grasping visual context |
Audio | Transcription, extracting meaning |
Mixed Content | Cross-referenced insights |
AI systems now use attention mechanisms to boost accuracy in analyzing complex documents. This is especially useful for mixed content, like documents that combine printed and handwritten text, or cluttered visuals. In the legal field, for instance, AI can process thousands of contracts at once, pinpointing key clauses and identifying risks without losing the broader context.
"The integration of advanced deep learning techniques and Large Language Models has transformed unstructured document processing, enabling AI to focus on relevant content parts and achieve higher recognition accuracy, especially in challenging scenarios with noise or clutter."
The biggest strength of AI in this area is its ability to handle massive amounts of data while retaining context. If you're looking for tailored tools, BestofAI's database offers solutions designed for tackling even the most complex unstructured data challenges.
Efforts are now centered on refining contextual understanding and addressing ethical and privacy concerns, as managing unstructured data at scale continues to pose challenges.
Examples of AI in Contextual Data Processing
In healthcare, AI systems are used to analyze electronic health records (EHRs) while preserving critical data relationships. This allows healthcare providers to create more precise treatment plans by factoring in a patient’s medical history, current conditions, and external influences.
Industry | Implementation | Impact |
Healthcare | EHR Analysis | Better predictions for patient outcomes |
Finance | Market Analysis | Improved detection of anomalies |
Cybersecurity | Network Monitoring | Real-time identification of threats |
The financial and cybersecurity sectors have also seen major progress through the use of AI. For instance, contextual AI is enhancing financial recommender systems by combining market data with broader economic indicators. This results in more refined investment suggestions. In cybersecurity, AI tools analyze network traffic to detect potential threats, distinguishing between normal activity and suspicious patterns based on historical data.
"The integration of context-aware AI algorithms in financial recommender systems has demonstrated superior performance in predicting market trends and identifying investment opportunities compared to traditional analysis methods." - Venkatachalam Parvathy and Ray Sanjog
Even smaller organizations and startups are now able to access AI tools. Platforms like BestofAI help businesses discover specialized tools with ratings and user reviews, making advanced contextual processing more attainable for companies of all sizes.
While these examples showcase AI’s practical uses across various industries, achieving contextual accuracy in real-time applications remains a challenge. Success depends on processing large volumes of data while accounting for timing and external factors that affect interpretation.
These applications underline AI’s potential but also highlight the hurdles involved in scaling and improving contextual analysis - topics we’ll dive into further in the next section.
Improving Cybersecurity
AI-driven cybersecurity tools are changing the game when it comes to spotting threats. By analyzing network activity, user behavior, and system events in real time, these systems can detect possible breaches before they turn into full-blown incidents. This real-time analysis is key to staying ahead of cyber threats.
Take phishing attacks, for example. AI can examine email content, sender behavior, and user interactions all at once to identify even the most subtle threats. If it spots unusual patterns - like emails sent at odd hours or slight changes in tone - it can automatically quarantine the message and notify the security team, protecting users before any harm is done.
"The integration of contextual AI in cybersecurity has transformed our ability to detect and respond to threats. We're now seeing response times measured in seconds rather than hours or days", - IBM Security's 2024 Cost of a Data Breach Report
Here’s how AI enhances cybersecurity:
Capability | Function | Impact |
Threat Detection & Intelligence | Monitors network behavior and global security data | Cuts false positives by 67%, detects threats 8x faster |
Automated Response | Launches immediate countermeasures | Speeds up breach containment by 74% |
AI's strength lies in analyzing unstructured data sources like system logs and email communications. This allows security teams to gain deeper insights into potential threats, leading to more precise assessments and tailored responses.
Better Healthcare Systems
In 2022, the University of California, San Francisco conducted a study that highlighted how AI is reshaping cardiovascular care. By analyzing medical images alongside patient data, their system achieved an impressive 85% accuracy in identifying high-risk patients. This shows how AI can handle complex medical information far better than traditional methods. Similarly, the Mayo Clinic reported in 2023 that their AI system led to a 20% drop in hospital readmissions.
Contextual AI takes things a step further by blending various data sources - like patient histories, genetic details, and real-time monitoring data. This allows healthcare providers to make decisions based on a complete picture of the patient, not just isolated symptoms. One area where this is especially impactful is personalized medicine, where treatment plans are designed around an individual's genetics, lifestyle, and medical history.
AI Application | Impact | Measurable Outcome |
Disease Prediction | Early detection of high-risk conditions | 85% accuracy in cardiovascular risk assessment (UCSF) |
Patient Monitoring | Real-time health status tracking | 20% reduction in readmissions (Mayo Clinic) |
Treatment Planning | Tailored therapies for individuals | Better patient outcomes through targeted treatments |
"AI has the potential to revolutionize healthcare by providing deeper insights into patient conditions and improving treatment outcomes." - Dr. Eric Topol, Director of the Scripps Research Translational Institute
AI systems are also being used to analyze unstructured medical data, such as imaging results, clinical notes, and sensor readings. These tools are particularly good at spotting patterns that might go unnoticed by human experts.
One of the biggest strengths of Contextual AI is its ability to continuously learn and improve. As it processes more patient data, its predictions and treatment suggestions become increasingly precise. This ensures that healthcare becomes more tailored and effective as time goes on.
While AI is making waves in healthcare, its influence is also being felt in other critical fields like finance and cybersecurity.
Applications in Finance
AI's ability to analyze unstructured data and spot irregularities has led to major advancements in the financial world, particularly in fraud detection, trading, and risk assessment. For instance, Stripe has developed a fraud prevention system that examines device details, purchasing behavior, and location data, achieving an impressive 92% accuracy in identifying fraudulent transactions.
Big names like JPMorgan Chase and Goldman Sachs are also making strides with AI. JPMorgan has improved fraud detection by 35%, while Goldman Sachs has increased trading accuracy by 28%. These improvements come from using AI to analyze real-time data, including economic trends, news sentiment, and even social media activity, giving them a more detailed understanding of market dynamics.
Morgan Stanley showcases how AI can transform risk assessment. Their system reviews regulatory documents, news, and market reports, cutting evaluation time by 40% without sacrificing accuracy.
"AI's ability to process contextual data in finance isn't just about speed – it's about understanding the complex interplay between market conditions, regulatory changes, and global events."
AI isn't just for the financial giants. Fintech startups are also creating specialized tools to tackle unique challenges in the industry. Across the board, financial institutions are ramping up their AI investments, with 70% planning to boost their capabilities by 2025. These efforts focus on analyzing diverse financial data while staying compliant with regulations.
As AI continues to grow, its impact on finance highlights both its promise and the complexities of expanding such technologies across various sectors.
Challenges and Future of Contextual AI
As AI continues to reshape how we process contextual data, organizations are encountering a range of challenges. One of the biggest hurdles is managing the overwhelming variety of data streams. Organizations are dealing with both structured and unstructured data, and the task of integrating this information effectively is no small feat. The sheer scale and diversity of the data demand AI systems capable of handling multiple data types with speed and precision.
Privacy and ethics are also major concerns in the development of contextual AI. Companies need to strike a balance between thorough data analysis and respecting individual privacy. To address this, many are turning to advanced solutions like encryption and differential privacy techniques. These methods allow for real-time data analysis while keeping sensitive information secure.
The integration of AI with data science is moving forward quickly, especially in areas that demand instant contextual understanding. Advances in deep learning and large language models are making it possible to analyze unstructured documents more effectively, leading to better insights and predictions.
Looking ahead, contextual AI is expected to evolve in several important areas:
Trend | Impact | Challenge |
Deep Learning Integration | Better data processing | High computing demands |
Real-time Analysis | Quicker decision-making | Ensuring data quality |
Privacy-focused AI | Improved regulatory compliance | Technical hurdles |
Contextual Understanding | Enhanced pattern recognition and semantic analysis | Transparency in algorithms |
Future systems will need to address challenges like handling unstructured data and maintaining ethical practices. As these obstacles are overcome, contextual AI is set to reshape industries, opening up new possibilities and driving progress across various sectors.
Managing Large and Diverse Data
Organizations today are dealing with the challenge of managing enormous amounts of information in various formats. AI systems have stepped in to tackle this issue by using advanced methods to integrate and process both structured data (like database entries) and unstructured content (such as social media posts, images, or sensor data) in real-time.
AI-powered analytics are making a big impact in industries like financial services. These platforms can process market data, news feeds, and transaction records all at once, helping detect fraud or uncover trading opportunities. In healthcare, AI systems analyze patient records, medical images, and real-time monitoring data to assist in making better clinical decisions.
Large Language Models (LLMs) have changed the game when it comes to processing documents. For example, in legal departments, LLMs can review thousands of contracts and regulatory documents at the same time, identifying compliance requirements and risks without losing the overall context.
AI's ability to manage diverse data effectively comes down to understanding context across various formats, including:
Natural Language Processing (NLP) for analyzing text and documents
Computer Vision for interpreting visual content
Deep Learning Models for recognizing patterns in time-series data
Context-Aware Algorithms for analyzing behaviors
"The integration of AI in contextual data processing has become a cornerstone for organizations seeking to make informed decisions. The ability to process diverse data types while maintaining context has transformed how we extract value from our data assets."
To handle large and varied datasets effectively, organizations should prioritize:
Building scalable systems: Invest in infrastructure that can process multiple types of data at once.
Ensuring data quality: Validate data consistently across all sources to maintain accuracy.
Using AI-driven analytics: Apply advanced algorithms to gain a deeper understanding of contextual data.
Combining these strategies helps organizations manage the complexities of modern data environments while uncovering insights that can fuel business growth.
As organizations improve their ability to handle diverse datasets, attention is shifting toward addressing ethical and privacy issues in AI-driven data processing.
Privacy and Ethical Concerns
As AI technology becomes more advanced in analyzing and interpreting contextual data, organizations are facing growing challenges in safeguarding privacy and adhering to ethical standards. These issues are especially pressing in fields like healthcare, finance, and cybersecurity, where AI systems are playing a larger role in critical decision-making.
Leading tech companies are taking steps to address these concerns through well-defined frameworks. For example, Microsoft has implemented AI principles aimed at ensuring transparency and accountability when handling sensitive data that might reveal personal details or behavioral patterns.
However, AI systems can unintentionally reinforce biases, particularly when they process contextual information for decision-making in areas like employment, healthcare, and finance. This raises serious concerns about fairness and accountability in these AI-driven processes.
"The integration of AI technologies into existing processes requires a deep understanding of the ethical and privacy implications." - Dr. Alexandra Mateescu, Researcher at Data & Society
To tackle these challenges, organizations should consider several safeguards:
Data Minimization: Collect and process only the data necessary for the specific purpose.
Transparency Protocols: Clearly communicate how AI systems use and interpret data.
Regular Audits: Frequently review AI systems to identify and address biases or privacy risks.
Regulations like GDPR in Europe and CCPA in California emphasize the urgent need for strong privacy and ethical protections. These include measures such as obtaining proper consent and ensuring individuals retain control over their personal data.
Privacy Challenge | Ethical Concern | Recommended Action |
Data Collection | Informed Consent | Adopt clear and transparent policies |
Algorithm Bias | Fairness | Conduct regular bias testing and fixes |
Data Security | Protection | Strengthen encryption and access controls |
Transparency | Accountability | Document AI decision-making processes |
Organizations working with contextual data must engage stakeholders and establish clear ethical guidelines. This involves providing regular training for teams managing AI systems and creating frameworks that promote responsible use while safeguarding individual privacy.
Striking the right balance between privacy and ethics in AI isn't just a technical issue; it's a societal challenge. It demands ongoing collaboration and innovation. As AI continues to advance, maintaining this balance is essential for building trust and ensuring progress in the field.
New Trends in Contextual AI
Explainable AI (XAI) is becoming a key technology, making AI decision-making more transparent and easier to understand. This transparency is essential for creating trust and clarity in AI systems used across various industries.
Systems using adaptive models and hybrid AI approaches are making strides in contextual AI. These technologies adjust their behavior based on changing conditions and combine different AI methods for more refined processing. For example, in e-commerce, adaptive models update product recommendations in real-time, responding to user behavior and shifting market trends.
"The integration of AI and data science requires careful consideration of context, including technical, humanistic, ethical, political, and economic factors, to ensure effective and responsible use of AI technologies."
Another major development is the rise of bottom-up AI methodologies. These systems build their understanding from detailed, granular data points, transforming how information is processed and interpreted. By analyzing patterns and relationships at a fundamental level, organizations can generate valuable insights from complex data.
Here’s a quick look at some emerging trends in contextual AI:
Trend | Impact | Application Areas |
Explainable AI | Greater transparency in decision-making | Manufacturing, Healthcare, Finance |
Adaptive & Context-Aware Systems | Personalized and dynamic responses | Real-time analytics, User Experience |
Bottom-up AI Methodologies | Better pattern recognition and insights | Document analysis, Natural Language Processing |
These technologies are enabling systems to manage multiple layers of context at once, resulting in more advanced and precise analysis in complex scenarios.
Looking ahead, contextual AI is moving toward systems that can process massive datasets while staying accurate and relevant. This evolution reflects the growing demand for AI tools that can adapt to intricate, real-world environments while being transparent and accountable in their operations.
However, tapping into the full potential of these advancements comes with its own set of challenges, which organizations must carefully navigate.
Conclusion
AI has transformed how organizations handle contextual data, making it possible to uncover deeper insights from intricate data sets using advanced algorithms and machine learning. One standout example is the PINNACLE geometric deep learning model, which has set new standards in therapeutic target prediction.
The PINNACLE model has shown impressive results, particularly in analyzing conditions like rheumatoid arthritis and inflammatory bowel disease. This demonstrates how AI can navigate and make sense of complex biological data while staying contextually accurate.
Here are some key advancements shaping the future of contextual AI:
Development | Impact | Application |
Context-Aware Processing | Combines data from various sources for better decisions | Healthcare diagnostics and treatment plans |
Predictive Analytics | Uses historical trends to forecast future outcomes | Supply chain management |
Real-time Contextual Analytics | Adapts quickly to changing conditions | Financial market analysis and risk control |
Looking ahead, AI's role in contextual data processing is only growing. Tools like BestofAI, which offers a massive database of over 12,592 AI tools, highlight the vast array of options available for tackling complex challenges.
As organizations scale these technologies, maintaining precision and ethical responsibility will be essential. Balancing innovation with accountability will be key to unlocking the full potential of contextual AI.
Main Points to Remember
"Context is so vital that... DSiC includes the word in its title to emphasize the importance of four contextual dimensions affecting the practice of the field."
AI has brought a new level of precision to handling contextual data, with the PINNACLE geometric deep learning model leading the way in predicting and analyzing therapeutic targets across various cell types.
Here’s a look at how AI is reshaping contextual data processing:
Capability | Impact | Real-World Application |
Unstructured Data Processing | Transforms raw data into actionable insights | AI interpreting medical imaging, clinical notes, and lab results |
Context Integration | Merges diverse data sources for deeper analysis | Multi-dimensional analysis of cell types |
Real-time Analytics | Supports immediate, data-driven decisions | High-frequency trading and market risk assessments |
Scalable Processing | Efficiently manages vast, complex datasets | Analyzing health data across entire populations |
These developments underscore the importance of choosing the right tools and frameworks to fully leverage contextual AI. Advanced AI systems excel at processing unstructured data while factoring in key contextual details like time, location, and user behavior. This is especially impactful in healthcare, where such analysis provides a more complete picture, improving patient outcomes.
For organizations exploring contextual AI, platforms like BestofAI offer access to over 12,592 specialized tools spanning various categories. It’s critical for organizations to focus on solutions that balance innovation with ethical practices, ensuring AI is deployed responsibly while maximizing its analytical strengths.
Using BestofAI to Find AI Tools
BestofAI provides access to a database of over 12,592 AI tools, neatly organized into categories to help users locate solutions for contextual data processing.
When searching for contextual data processing tools, focus on these key areas:
Category | What to Look For | Why It Matters |
Data Processing | Tools with real-time analytics | Enables quick, context-aware decisions |
Context Analysis | Natural language understanding | Helps interpret unstructured data |
Integration Tools | API compatibility | Ensures smooth integration with systems |
To refine your search, take advantage of the platform's sorting options, which let you filter tools by popularity or user ratings. For instance, within the real-time analytics category, you can find tools designed for tasks like processing live data streams or recognizing behavioral patterns. You can also contribute by submitting new tools, helping the platform stay updated with the latest AI innovations.
For businesses looking to implement contextual data processing systems, BestofAI’s categorized structure makes it easier to pinpoint the right tools. Whether the goal is handling unstructured data, analyzing behaviors, or incorporating time-sensitive factors, platforms like BestofAI simplify the process of finding the right AI solutions for operational needs.