Lilac
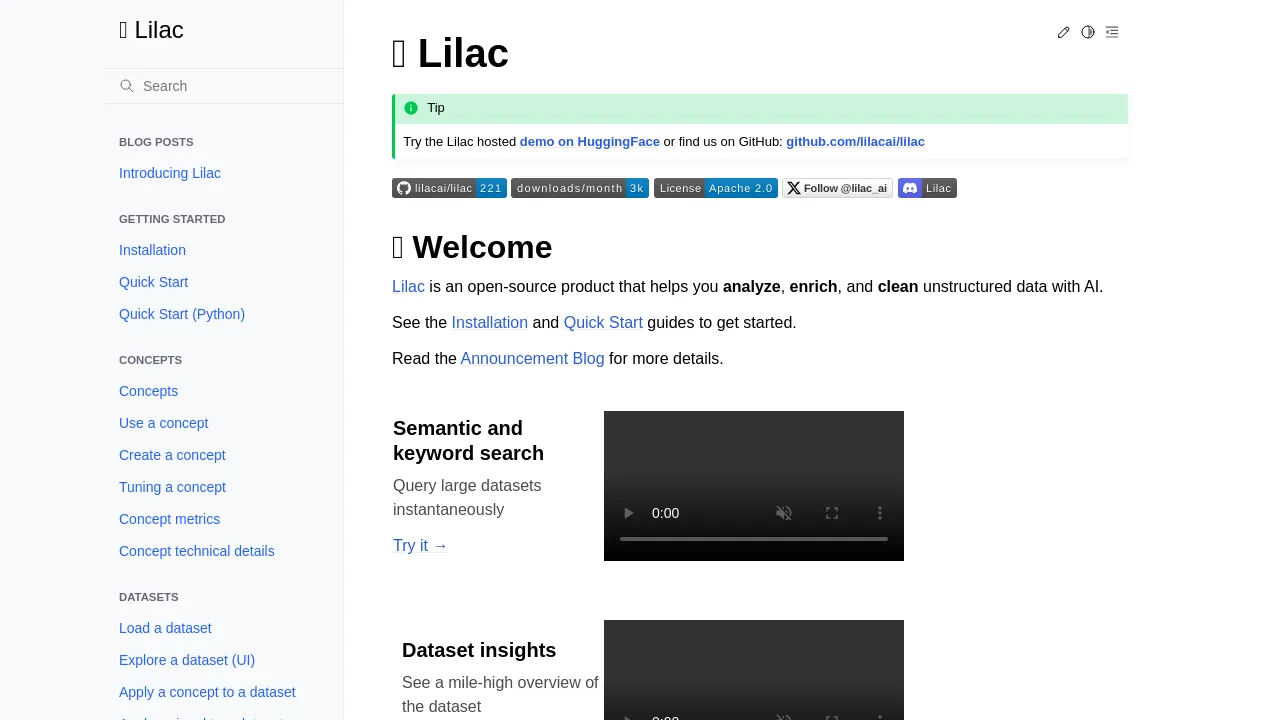
Lilac Overview
Lilac is an open-source AI product designed to analyze, enrich, and clean unstructured data. It provides a visual tool and a Python API to explore datasets with natural language, enrich datasets with metadata, conceptually search and tag data, remove unwanted data based on user-defined criteria, and analyze patterns in data. Lilac operates entirely on device using robust open-source LLM technologies.
Lilac Highlights
- Lilac allows users to explore datasets with natural language, making it a powerful tool for handling documents and other text-based data.
- It provides the ability to enrich datasets with metadata, including PII detection, profanity, text statistics, and more.
- Lilac offers a unique feature to conceptually search and tag data, such as finding paragraphs about a specific topic like injury.
Use Cases
A market research company uses Lilac to clean and analyze unstructured data collected from various sources like social media, customer reviews, and surveys. The company uses the natural language exploration feature to understand the sentiments and opinions of their target audience. They also use the metadata enrichment feature to categorize data based on PII detection, profanity, and text statistics.
The company is able to gain valuable insights about their target audience, which helps them in making informed business decisions. The cleaned and enriched data also improves the accuracy of their research findings.
A law firm uses Lilac to search and tag data in their vast repository of legal documents. They use the conceptual search feature to find paragraphs about specific topics like injury, property disputes, or corporate law. This helps them quickly locate relevant information without having to read through entire documents.
The law firm is able to save time and increase productivity by quickly finding relevant information. This also improves their ability to provide accurate and timely legal advice to their clients.
A journalist uses Lilac to enrich datasets with metadata for investigative reporting. They use the metadata enrichment feature to add context to their data, such as identifying PII, detecting profanity, and analyzing text statistics. This helps them understand the data better and write more comprehensive reports.
The journalist is able to write more in-depth and accurate reports based on the enriched data. This improves the quality of their reporting and increases their credibility.