Markovml
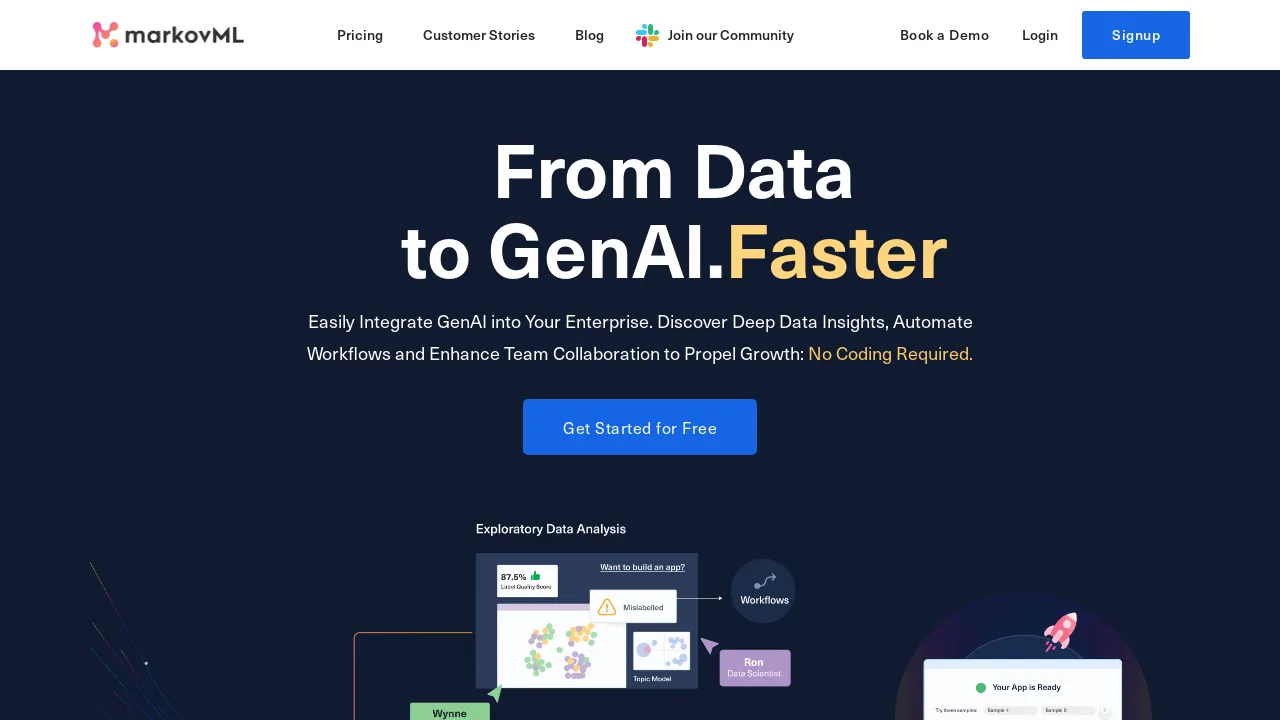
MarkovML Overview
MarkovML is a powerful AI tool designed to streamline data management and analysis, and enhance team collaboration. It offers a no-code GenAI app builder, AI evaluators, and a collaborative tool called Snippet. The GenAI app builder empowers domain experts to create GenAI apps for a variety of use cases. The AI evaluators help evaluate machine learning models on cost, business impact, and bias. Snippet is a collaborative tool designed for AI projects, bringing team knowledge, ideas, metrics, analysis, feedback, visualization, and more in a shared workspace. MarkovML also ensures robust data governance, privacy, and security.
MarkovML Highlights
- Intuitive no-code GenAI app builder for creating a variety of AI applications.
- State-of-the-art AI evaluators for assessing machine learning models on cost, business impact, and bias.
- Snippet, a collaborative tool designed for AI projects, enhancing team collaboration and alignment.
Use Cases
A project manager in a tech company uses Snippet to enhance team collaboration on an AI project. The tool is used to share knowledge, ideas, metrics, analysis, feedback, and visualizations in a shared workspace.
The team is able to work more effectively and efficiently on the AI project. This leads to a higher quality end product and a shorter project timeline.
A domain expert in a retail company uses the no-code GenAI app builder to create a custom AI application. The application is designed to predict customer buying behavior based on historical purchase data and demographic information.
The retail company is able to implement a more effective marketing strategy based on the predictions made by the AI application. This leads to increased sales and customer satisfaction.
A data scientist in a healthcare organization uses the AI evaluators to assess a machine learning model designed to predict patient readmission rates. The evaluators are used to measure the cost, business impact, and potential bias of the model.
The healthcare organization is able to identify any potential issues with the model before it is implemented. This allows them to make necessary adjustments to improve the model's accuracy and fairness, ultimately leading to better patient care.